Dr. Zuqi Tang
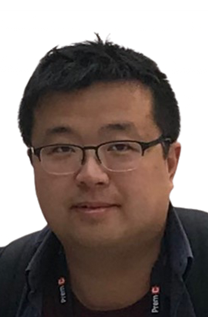
Zuqi Tang is an Associate Professor in the Department of Electrical Engineering at the University of Lille, France. He received his B.S. in Pure Mathematics from Wuhan University, China, in 2007, followed by an M.S. in Applied Mathematics in 2009 and a Ph.D. in Electrical Engineering in 2012, both from the University of Lille 1. After completing postdoctoral fellowships at Électricité de France (EDF) and INRIA Paris-Rocquencourt (2012–2015), he served as an Assistant Researcher at CNRS (2015–2017). Since 2017, he has held a tenured position at the University of Lille, and in 2023, he obtained the French Habilitation à Diriger des Recherches (HDR), which qualifies him to supervise doctoral research.
His research focuses on numerical modeling and analysis in computational electromagnetism, with a recent emphasis on developing numerical tools for the Darwin model and exploring various numerical techniques in the context of digital twins, particularly the application of deep learning techniques in surrogate model construction.
Presentation: Towards purely parameter-specific surrogate model construction in the context of digital twins: a tensor decomposition-based approach
In the context of digital twins, surrogate model reconstruction is essential for real-time control, fault diagnosis, and performance prediction. While data-driven techniques are widely used, their accuracy heavily depends on the availability of large, high-quality datasets. In contrast, projection-based model order reduction (MOR) techniques, such as Proper Orthogonal Decomposition (POD), preserve the structure of the physical model, ensuring greater interpretability. However, these methods often lack robustness when handling multiple varying parameters, which can compromise their accuracy and reliability in multi-parameter electromagnetic problems. To address these challenges, a hybrid approach leveraging tensor decomposition has recently been proposed. This method enables the identification of an optimal low-dimensional representation of the parametric problem, thereby enhancing the robustness of surrogate models in both data-driven and model-driven techniques. Applied to multi-parameter electromagnetic problems across various scenarios, the approach has demonstrated superior performance compared to classical methods. These results highlight its potential for advancing digital twin applications in electrical engineering.